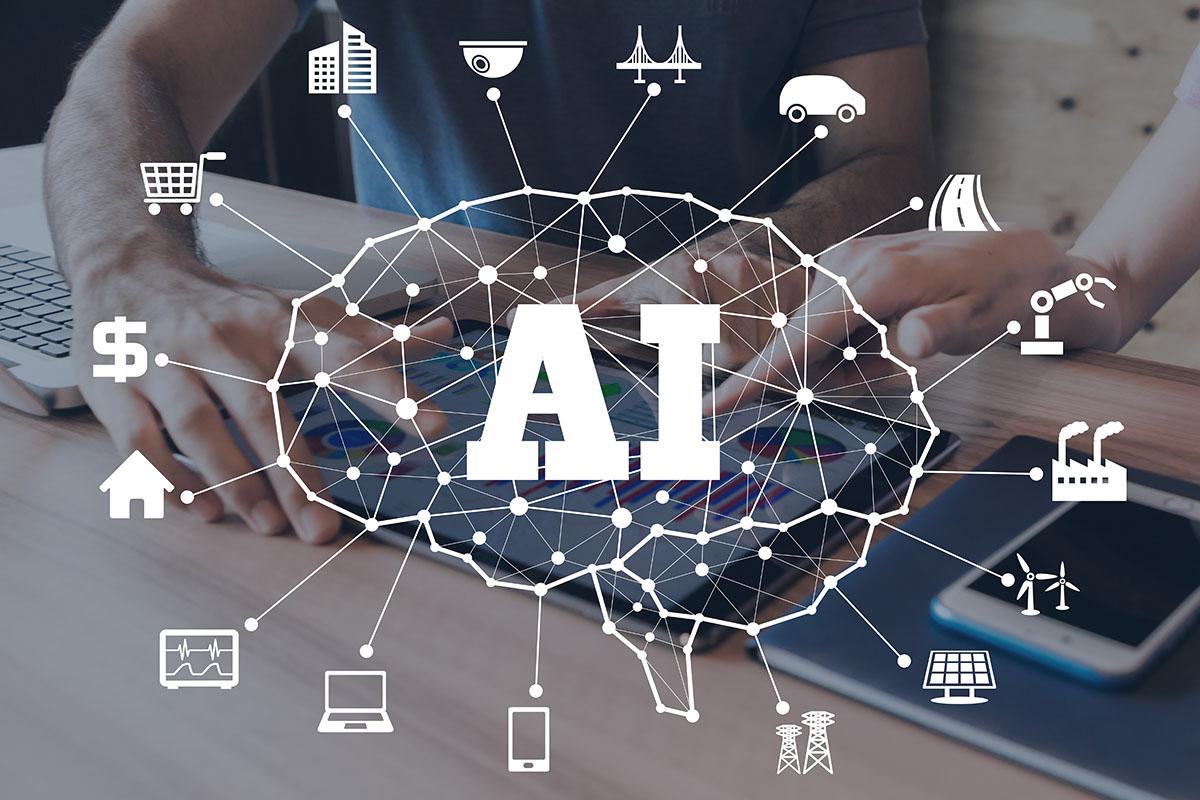
In the fast-paced world of artificial intelligence, staying safe is a top priority. New guidelines on AI security are changing the game for developers and users alike. The US and UK have jointly released guidelines for secure AI system development, which provide essential recommendations for AI system development and emphasize the importance of adhering to Secure by Design principles.
Whether you're a tech whiz or just curious, understanding these guidelines is critical to navigating the exciting world of AI. From protecting your data to making sure AI is used ethically, we'll explore how these rules impact the way we create and interact with AI. In this article, we will discuss what do the new AI security guidelines mean for developers and users.
Importance Of AI Model Development In A Secure Environment
As artificial intelligence keeps transforming industries and business processes, it becomes increasingly important to make sure AI models are secure. Organizations may reliably deploy AI models that provide value while limiting risks associated with unauthorized access and breaches by emphasizing security right from the start.
It is essential to train, develop, and implement AI models in a safe environment for the following reasons:
Protecting Sensitive Data
Large volumes of data, such as Personally Identifiable Information (PII), financial records, medical records, or confidential company information, are frequently needed for AI models to be trained.
This data is sufficiently shielded from misuse, breaches, and unauthorized access thanks to a secure environment.
Preventing Data Tampering
One of the main factors influencing an AI model's performance is the quality of the training data. Malicious actors may alter or tamper with the data in an insecure training environment, producing biased models or compromised outcomes.
The environment's security contributes to the preservation of the training data's integrity, guaranteeing the accuracy and equity of the AI models.
Safeguarding Intellectual Property
AI models frequently need significant time, energy, and resource commitments. The intellectual property linked to these models is better safeguarded in a secure environment, which deters theft, illegal access, and reproduction.
It guarantees that the company maintains ownership of its unique model designs, training regimens, and algorithms.
Mitigating Adversarial Attacks
Adversarial assaults, in which evil parties purposefully alter input data to trick or abuse the model, can weaken AI models.
Organizations may reduce the danger of exploitation and manipulation by implementing strong defenses against such attacks, such as input validation, anomaly detection, or model monitoring, by installing AI models in a safe environment.
Ensuring Regulatory Compliance
Sensitive data processing and protection are subject to stringent restrictions, including financial rules (PCI-DSS), industry-specific compliance frameworks (HIPAA for healthcare, for example), and data protection legislation (GDPR)
. Organizations may abide by these requirements and avoid the financial and legal ramifications of non-compliance by maintaining a secure environment.
Maintaining Trust And Reputation
Incidents using AI models or security breaches can have dire repercussions, undermining the organization's credibility and harming its brand. Organizations may exhibit their commitment to safeguarding data privacy, avoiding abuse, and guaranteeing the dependability and equity of their AI applications by placing a high priority on security across the whole lifetime of their AI models. This promotes trust among stakeholders, consumers, and users.
New AI Security Guidelines For Secure Design
Raise Staff Awareness Of Threats And Risks
Ensuring the security of AI systems starts with raising awareness among staff, including system owners, senior leaders, data scientists, and developers.
Training programs should cover relevant security threats, failure modes, and best practices in secure coding. Additionally, users should be educated about the unique security risks associated with AI systems.
Model The Threats To Your System
An essential aspect of secure design involves conducting a comprehensive threat modeling process.
This includes assessing potential impacts on the system, users, organizations, and society in the event of AI component compromise. Sensitivity and types of data used in the system should be considered, recognizing that AI systems can be attractive targets for attackers.
Design Your System For Security
The design phase must prioritize security alongside functionality and performance. Factors such as supply chain security, model choices, and user interaction should be carefully considered.
Secure development and operation practices, including least privilege principles, should be integrated into the AI system development process.
Consider Security Benefits And Trade-Offs When Selecting Your AI Model
Choosing the suitable AI model involves balancing various requirements. Factors such as model complexity, appropriateness for the use case, interpretability, and characteristics of the training dataset must be considered. Regular reassessment based on evolving security research is crucial.
New AI Security Guidelines For Secure Development
Secure Your Supply Chain
Developers should assess and monitor the security of AI supply chains throughout the system's life cycle. Suppliers must adhere to the organization's security standards, and alternate solutions should be considered for mission-critical systems if security criteria are not met. Guidance from resources like the NCSC's Supply Chain Guidance should be followed.
Identify, Track, And Protect Your Assets
Understanding the value of AI-related assets and implementing processes to track, authenticate, version control, and secure these assets is crucial. Technical debt, a common challenge in AI development, should be managed throughout the life cycle, considering rapid development cycles and evolving protocols.
Document Your Data, Models, And Prompts
Comprehensive documentation of models, datasets, and prompts is essential for transparency and accountability. Documentation should include security-relevant information such as training data sources, scope, limitations, guardrails, cryptographic hashes or signatures, retention time, and potential failure modes.
Manage Your Technical Debt
Recognizing the challenges of managing technical debt in AI development, a proactive approach is required. Life cycle plans should assess, acknowledge, and mitigate risks associated with future similar systems.
New AI Security Guidelines For Secure Deployment
Secure Your Infrastructure
Applying sound infrastructure security principles across the system's life cycle is essential. Access controls for APIs, models, and data should be implemented, and environments holding sensitive code or data should be appropriately segregated.
Protect Your Model Continuously
Implementing standard cybersecurity best practices and controls on the query interface is crucial to protect the model from direct and indirect access. Cryptographic hashes and signatures should be used to validate models, ensuring their integrity.
Develop Incident Management Procedures
Incident response, escalation, and remediation plans should be in place, reflecting different scenarios and evolving research. Critical company digital resources should be stored in offline backups, and audit logs should be provided to customers to aid in their incident response processes.
Release AI Responsibly
Models, applications, or systems should only be released after undergoing appropriate security evaluation, including benchmarking and red teaming. Users should be informed about known limitations or potential failure modes, and settings should be configured to prevent malicious use.
New AI Security Guidelines For Secure Operation And Maintenance
Monitor Your System's Behavior
Continuous monitoring of model outputs and system performance is crucial for identifying security issues, intrusions, compromises, and natural data drift.
Monitor Your System's Inputs
In compliance with privacy and data protection requirements, monitoring and logging inputs to the system should be implemented. This includes explicit detection of out-of-distribution and adversarial inputs.
Follow A Secure By Design Approach To Updates
Automated updates, secure modular update procedures, and support for users to evaluate and respond to model changes are essential. Changes to data, models, or prompts should be treated with caution, and significant updates should be approached with the same diligence as new versions.
Collect And Share Lessons Learned
Participating in information-sharing communities and maintaining open lines of communication for feedback on system security is vital. Collaborating across the global ecosystem helps share best practices and quickly address vulnerabilities.
What Is The Impact Of Security Breaches On AI Models?
As businesses depend more and more on AI models to inform decisions and provide insights, it is critical to make sure these models are secure. Security lapses in AI models can have serious repercussions, such as lost financial gains, reputational harm, and compromised data integrity.
Compromised Data Integrity And Confidentiality
An AI model's security flaw might jeopardize the confidentiality and integrity of the data. Attackers may get sensitive data without authorization, alter it, or take useful information for nefarious ends. The accuracy and dependability of AI models are compromised by this data integrity violation, which results in inaccurate outputs and poor decision-making.
In addition, breached data confidentiality is against privacy laws and undermines consumer confidence, which can have a permanent negative impact on a company's reputation.
Intellectual Property (IP) Theft And Competitive Disadvantage
AI models frequently include trade secrets, exclusive algorithms, and creative methods created by businesses. IP theft may result from a security breach that makes these priceless intellectual properties available to unauthorized parties.
Intellectual property theft reduces market distinctiveness and income potential by undermining an organization's competitive edge and making it easier for rivals to copy or even outperform its AI models.
Financial Losses And Legal Liabilities
Organizations may face substantial financial losses and legal repercussions as a result of security breaches in AI models. The expenses linked to looking into and fixing a breach, alerting the parties involved, adding security, and restoring confidence may add up.
Groups may also be subject to legal action and fines from people or groups impacted by the breach. The monetary and legal repercussions may significantly impact the bottom line and long-term survival of a business.
Reputational Damage And Loss Of Customer Trust
The consequences of an AI model security breach can seriously harm an organization's reputation. They have publicized security lapses that damage consumer confidence since people are reluctant to use AI-powered services or provide their personal information.
An organization's brand image may be damaged by the bad press and media attention that accompanies a breach, making it difficult to win back customer trust. After a breach, restoring confidence takes a lot of time and money, which further affects an organization's ability to operate and compete.
What Is The Meaning Of New AI Security Guidelines For Developers And Users?
New AI security guidelines have been introduced to provide developers with a comprehensive framework for making informed decisions across the entire lifecycle of AI systems.
- Global Collaboration for Robust Guidelines- The guidelines are a result of collaborative efforts involving 22 security agencies worldwide and contributions from major players in the AI landscape, including Amazon, Google, IBM, Microsoft, OpenAI, and Palantir.
- Secure-by-Design and Secure-by-Default Principles- Emphasizing a proactive stance, the guidelines advocate for the implementation of "secure-by-design" and "secure-by-default" principles. Developers are urged to integrate security measures into AI products at the design stage, protecting them from potential attacks.
- Prioritizing Security Throughout Development- Developers are encouraged to prioritize security alongside functionality and performance throughout the decision-making process. This includes considerations like model architecture and training datasets. Setting the most secure options as defaults, with clear communication about alternative configurations, is a key recommendation.
- A Global Understanding of Cyber Risks- The guidelines mark a significant step in establishing a shared global understanding of cyber risks and mitigation strategies related to AI. Security is positioned as a core requirement throughout development rather than an afterthought.
- International Endorsement - Endorsed by 18 countries, including influential players like the United States and the United Kingdom, the guidelines represent a united front in recognizing the importance of incorporating security measures into AI development.
- Non-Binding but Historic Agreement - While the guidelines are not legally binding, they signify a historic agreement. Developers are urged to invest in these guidelines, making security an integral part of each step in system design and development.
AI Security Guidelines Implications For Users
For users, the new AI security guidelines mean increased assurance that AI systems are designed and developed with security as a priority. This proactive approach aims to reduce the risk of cyber-attacks and data breaches, emphasizing the shared responsibility of developers and users in maintaining a secure AI environment.
However, users are reminded to take personal steps, such as using strong passwords and staying vigilant online, to protect their data and privacy.
Frequently Asked Questions
How Do Guidelines Address Technical Debt In AI Development?
Guidelines recommend a proactive approach to managing technical debt throughout the AI system's life cycle, acknowledging and mitigating risks associated with future similar systems.
What's The User Benefit From AI Security Guidelines?
Users benefit from increased assurance that AI systems prioritize security, reducing the risk of cyber-attacks and data breaches while emphasizing the shared responsibility of developers and users.
What's The Impact Of Security Breaches On AI Models?
Security breaches in AI models can lead to compromised data integrity, intellectual property theft, financial losses, and reputational damage.
Everything Considered
In the end, here is the answer to your question about what do the new AI security guidelines mean for developers and users. The new AI security guidelines represent a comprehensive framework for developers and users to navigate the complex landscape of secure AI system development.
By addressing critical aspects of the development life cycle, from design to operation and maintenance, these guidelines aim to reduce the overall risk associated with AI systems. Adherence to these guidelines not only enhances the security posture of AI systems but also fosters a culture of responsible AI development.
As AI continues to play an increasingly integral role in various sectors, these guidelines serve as a vital resource for ensuring the ethical, secure, and transparent deployment of AI technologies. Developers and organizations are encouraged to incorporate these guidelines into their AI development practices, fostering a secure and resilient AI ecosystem.